Finding a Better Method for Early Autism Diagnosis
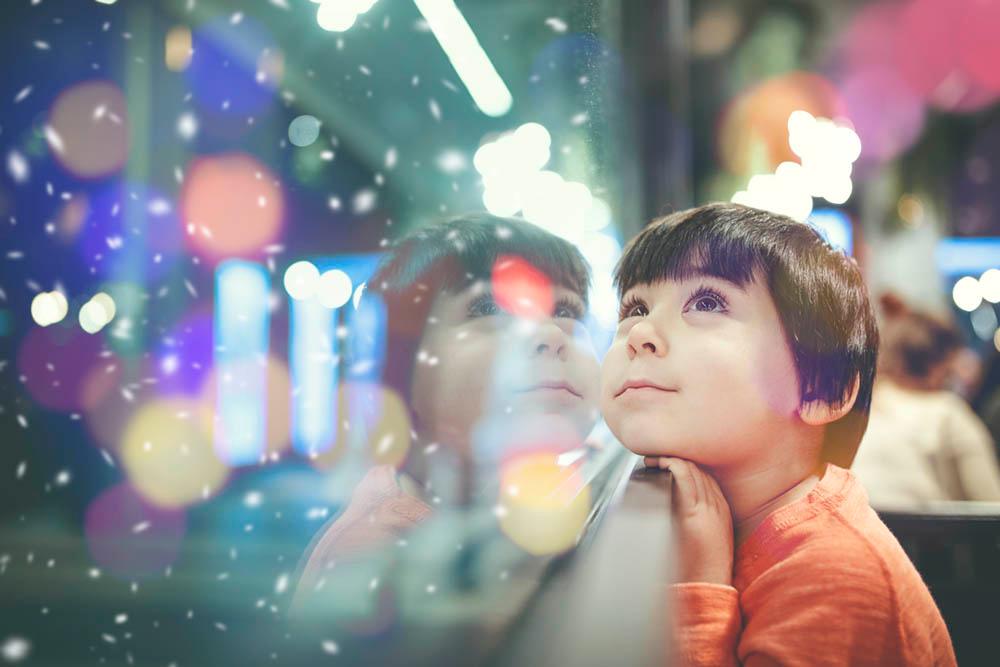
Even though symptoms of autism spectrum disorder can be apparent in children under a year old, kids aren’t diagnosed, on average, until they’re four and a half. Earlier diagnosis and treatment can improve an autistic child’s development and health outcomes, so researchers at Duke University Health System are piloting a program that uses machine learning and patient data from Epic to identify infants and toddlers at risk for autism and other neurodevelopmental disorders, such as attention deficit hyperactivity disorder (ADHD).
“If we can detect autism early and provide treatment, many children do very well,” said Dr. Geraldine Dawson, director of Duke’s Center for Autism and Brain Development and one of the program’s lead researchers.
Early intervention is important because younger children have higher neuroplasticity and are more likely to improve language and social skills.
The pilot assesses risk factors from kids’ medical records in Epic, such as premature birth, maternal complications during pregnancy, and delayed motor activity. Researchers will then use this data to develop an algorithm for earlier identification and intervention. With so much data at their fingertips, researchers could also uncover previously unknown risk factors.
This project was initially supported by the Forge, Duke University’s center for health data science, and was awarded an NIMH RO1 research grant.
“It’s a huge commitment,” said Dr. Scott Kollins, a professor of psychiatry and behavioral sciences in the School of Medicine at Duke and the other lead researcher on the pilot. “But we can’t not do this.”
Read more from Duke.